Women of Aurora: Tiffany Matz
Meet our team | November 21, 2019 | 5 min. read
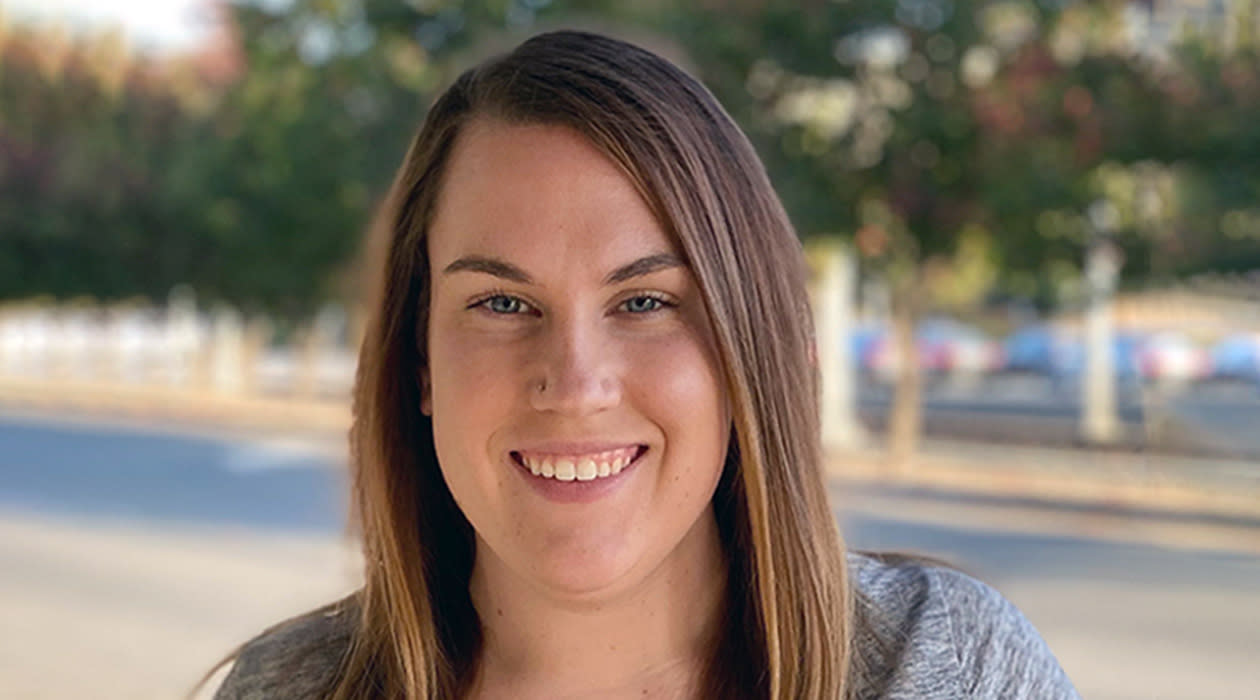
Working with the local motorcycle club Iron Order, Tiffany and our Vehicle Operations team set up a day of riding to collect data about how our cars see motorcycles in different types of complex situations.
How do you train a self-driving car to safely ride alongside motorcycles? One of the first steps involves our perception system, which analyzes sensor data and generates a representation of all actors around the vehicle, including motorcyclists and pedestrians. Making sure our cars “see” motorcycles properly is crucial as we train the Aurora Driver. Our machine learning models learn to recognize objects like motorcycles from incoming data from our radar, lidar, and cameras, but they can only do so if they have a good and diverse set of existing examples that are properly labeled. The better data we have, the better our models get at recognition.
Manual data collection is one part of a multi-prong approach that allows us to quickly get data for commonly-seen objects; we also use simulation and collect large amounts of data as we drive on the road.
Recently, we wanted to gather more data about motorcycles in a range of different and complex situations — moving at different speeds, approaching from different lanes, riding together, and more — to improve recognition performance. So we got a group of motorcyclists together for a day of riding and testing.
We sat down with Tiffany Matz from Vehicle Operations, which focuses on our on-road testing, to learn more about this project. She also shared what motivates her to work here and what she does in her free time (hint: COOKIES).
Tell us about your work at Aurora and what led you here. What motivates you and the team?
Tiffany: I’ve been at Aurora for more than two years. I am the Vehicle Operations Training Lead, which involves, as you might have guessed, a lot of training! I train our safety drivers to operate our vehicles and work with our technical teams as we develop the Aurora Driver.
I got into the industry a few years ago out of curiosity and fell in love with technology, research, and development. I feel like I am helping people by contributing to a technology that will make our roads safer.
The fact that Aurora’s various teams are all working towards a common goal — delivering the benefits of self-driving technology safely, quickly, and broadly — keeps everyone motivated. It’s rewarding to see how our team’s testing feedback directly helps improve the performance of the Aurora Driver.
You recently partnered with the local motorcycle club for a day of riding and data collection. What were you trying to learn?
Tiffany: We wanted to collect additional data on what motorcycles look like riding at different speeds and in different situations. This is valuable information and ensures our perception system properly recognizes motorcycles in all sorts of situations.
In this case, the request came directly from the Perception team, who told us, “Any scenario that mimics real-world car/motorcyclist interaction is worth getting data on.”
We were looking for as much information as possible in a variety of circumstances and partnering with the Iron Order motorcycle club allowed us to get a lot of diverse data quickly. For example, we varied:
-
positions relative to the car: including motorcycles in adjacent lanes, the same lane, in front of the car, and behind the car
-
approaches: including oncoming motorcycles, motorcycles passing the car, and motorcycles riding in front of the car
-
speeds: including scenarios where we ran the approaches listed above at various speeds, as well as scenarios where the car is stopped with a motorcycle in front of it
-
types of motorcycles: We ended up having an Indian, four Harleys, a KTM sports dirt bike, and a Yamaha cruiser bike.
Once you got the request, what happened next? How were you able to collect so much data in such a short time?
Tiffany: We wanted to act fast so we reached out to the Iron Order motorcycle club to get volunteers. We also had several Aurorans and an employee’s dad ride too!
Then we created the test plan — the number of motorcyclists expected, the Aurora vehicles needed, a list of specific scenarios we wanted to capture, and the mapped area that would allow us to capture those scenarios.
During the data collection, there was no need to drive in autonomous mode. Instead, our vehicle operators drove the cars in manual mode, which we call “manual data collections.” These collections have the added benefit of letting us capture how expert drivers handle these situations, data that is valuable when training our Driver.
Do you need to do manual collections like this every time you need more data?
Tiffany: We use a combination of different approaches when it comes to getting data for commonly seen objects. Manual data collection is one approach; simulation is another. We have a large data set that we collect out on the road, but as we identify areas where we want more data, we do targeted collections like this one. Our mission is to deliver the benefits of self-driving technology safely, quickly, and broadly. This speaks to the quickly part!
How did you make sure everyone was safe during this manual data collection?
Tiffany: Safety plays a large role in everything we do and this was no different. We had two safety operators in the car at all times, which is standard practice at Aurora, and we stuck to pre-mapped routes.
Preparation and communication are key. Before we hit the road, we held a safety briefing and reviewed the test plan. We went over the goals, the routes we’d be driving, and how each person would interact with the vehicle. The motorcyclists rode the route first to familiarize themselves with it. If anyone needed to stop for any reason, we established a hand signal to indicate that we should return to our meeting point.
How did it all turn out?
Tiffany: In addition to getting valuable data, this test gave us the chance to engage the public in our work. The Iron Order is obviously very invested in motorcycle safety, so we appreciated the opportunity to partner with them. We also saw a group of kids waving to us when we drove by and it was awesome to see their excitement.
It’s always a positive experience when we have the chance to include the community in our work. It allows us to show that no matter what your commuting style is — walking, biking, or driving — we care about the safety of everyone.
Here’s our vehicle out on the road, with a motorcycle behind it.
When you’re not working your day job, what is one of your favorite things to do?
Tiffany: I enjoy baking in my spare time! I love the mix of science and creativity. I just made coffee cake and brown butter chocolate chip cookies. I brought the treats into the office and they were a big hit. I also tried to bake my own bread. It didn’t go well but I am hoping to try it again soon.
What would you spend your time on if you didn’t have to spend your time driving to the office?
Tiffany: My commute can be quite long so I’d love to free up time to read, learn a new language, or finish up work before I get home.
What is the best thing about working at Aurora?
Tiffany: The entire company is really friendly and welcoming, with lots of cultural and social events that bring people together. And everyone has a voice when it comes to safety. That was really important to me and it speaks to the culture we’re building here.
Want to work on challenging problems like the ones described above? Aurora is delivering the benefits of self-driving technology safely, quickly, and broadly. We’re looking for talented people to join our team.
Delivering the benefits of self-driving technology safely, quickly, and broadly.