Capability spotlight: Prioritizing pedestrian safety
Aurora Driver | October 14, 2022 | 3 min. read
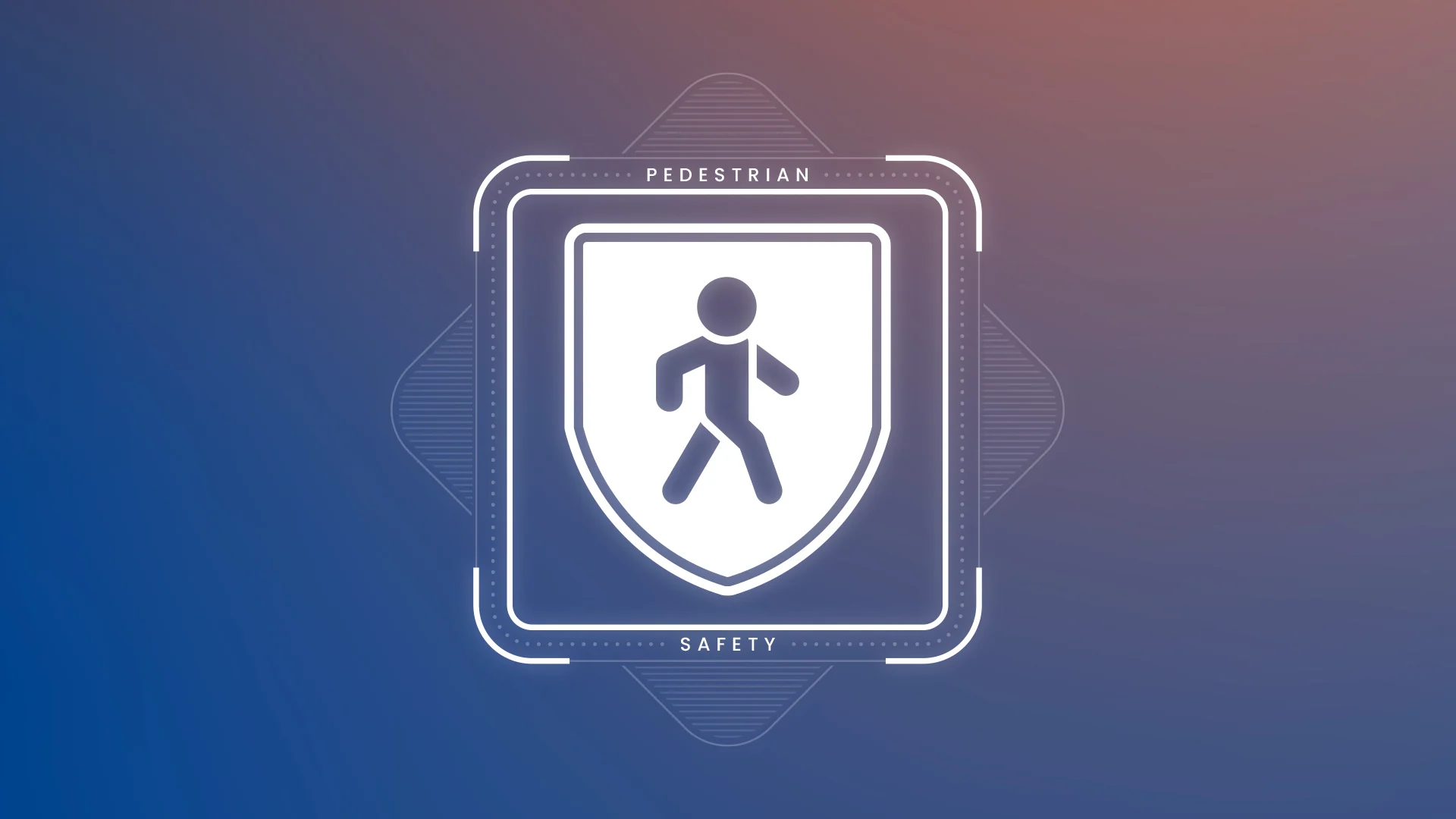
How we’re training the Aurora Driver to detect and safely respond to vulnerable road users
Even if you’ve never had to pull over and change a flat tire on the side of the highway, I’m sure you’d agree that it’s not a safe environment for a pedestrian. Cars fly by at 50-80 MPH, and depending on the width of the highway shoulder, you might not have much wiggle room between your poor car and a traffic lane.
When traveling at highway speeds, there is very little time for an Aurora Driver-powered truck or car to react to a pedestrian. Therefore, it is critical that the Aurora Driver is able to accurately and reliably detect vulnerable road users from hundreds of meters away.
What is a vulnerable road user?
We consider all pedestrians and people using human-powered means of transportation to be vulnerable road users. This can include someone changing a flat tire on the side of the road, a construction worker directing the flow of traffic, and even a law enforcement officer writing up a ticket. Off of the highway, it also includes the people who interact with Aurora Driver-powered vehicles, such as terminal operators and weigh station personnel, and anyone in the vehicle’s immediate vicinity, such as people crossing the street or biking to work.
Data collection
When the Aurora Driver is powering a truck or car on public roads, its primary objective is to complete its mission safely. This means the Aurora Driver must always be on the lookout for potentially unsafe situations, ready to proactively avoid them or mitigate them. To do this, the Aurora Driver relies on our purpose-built long-range perception system, made up of machine learning algorithms and a suite of camera, radar, and lidar sensors including our proprietary FirstLight Lidar.
To allow our perception system to reliably recognize and respond to all kinds of vulnerable road users in different situations, we are training it on high-quality datasets. We collect this data while hauling freight for our pilot customers, testing other new capabilities on the road, and conducting manual data-capturing missions.
As we’ve said many times before, construction is a year-round fixture on Texas highways. Our trucks frequently pass construction and work crews doing all sorts of things, providing us with valuable data of people hanging off of vehicles, partially occluded by equipment, bending over, holding signs, and more.
Drivers sometimes pull over onto the shoulder during these long highway stretches to take a break, make a quick repair, or call for a tow.
While testing on I-20 between Fort Worth and El Paso, we often pass Texas state troopers standing next to pulled-over vehicles on the side of the road.
Sometimes we pass pedestrians who are not accompanied by vehicles, such as someone wielding a leaf blower or picking up trash on a median.
Occasionally we come across brave souls walking dogs, jogging, or biking alongside interstate traffic.
To collect suburban and urban pedestrian data, we manually drive our Aurora Driver-enabled Toyota Siennas around Pittsburgh and Palo Alto. This gives us natural examples of people using crosswalks, entering parked cars, and hanging out on street corners at all times of day.
To augment the data we capture on the road, we also expose the Aurora Driver to rare or unusual data within safe, controlled environments such as our test tracks or office lots. In these targeted data collection efforts, we ask vulnerable road users to act out situations that are difficult to find out in the wild, while stationary Aurora Driver-powered vehicles record logs.
These logs of people moving around in different ways, wearing costumes, and holding various items allow us to test how different behaviors and changes in appearance affect perception performance.
Data of people using rollerskates, wheelchairs, skateboards, and different kinds of bikes allow us to train the Aurora Driver to perceive various forms of human-powered wheeled transport.
Children often behave in unpredictable ways and may not know how to safely use the road, so we’ve also held events where Aurora employees with children can volunteer to help us collect data to train the Aurora Driver to detect and track their movements.
Training and testing
As with all of the Aurora Driver’s autonomy capabilities, we are conducting dozens, hundreds, and sometimes thousands of virtual tests to teach the Aurora Driver to behave safely around vulnerable road users.
The real-world data we collect enters our machine learning data engine where we generate the ground truth and create training and testing datasets. It also feeds our offline testing framework, where we test how different Aurora Driver software releases perform against the scenarios in these real-world logs.
We recreate some of the data we collect in simulation—unlike real-world tests using dummies, the Aurora Driver perceives the simulated pedestrians in our virtual tests as real, producing results that are representative of what the Aurora Driver would do when faced with a similar scenario in the real world. This allows us to accurately test the Aurora Driver on scenarios that could be dangerous to test with real people.
We look forward to sharing progress updates in the coming months, as the Aurora Driver’s vulnerable road user handling in high-speed operation capability matures and we continue to work toward Feature Complete.
Senior Director, Perception