Preventing Fatal Collisions: How Autonomous Trucks Could Save Lives in the Golden State
Safety | August 27, 2024 | 3 min. read
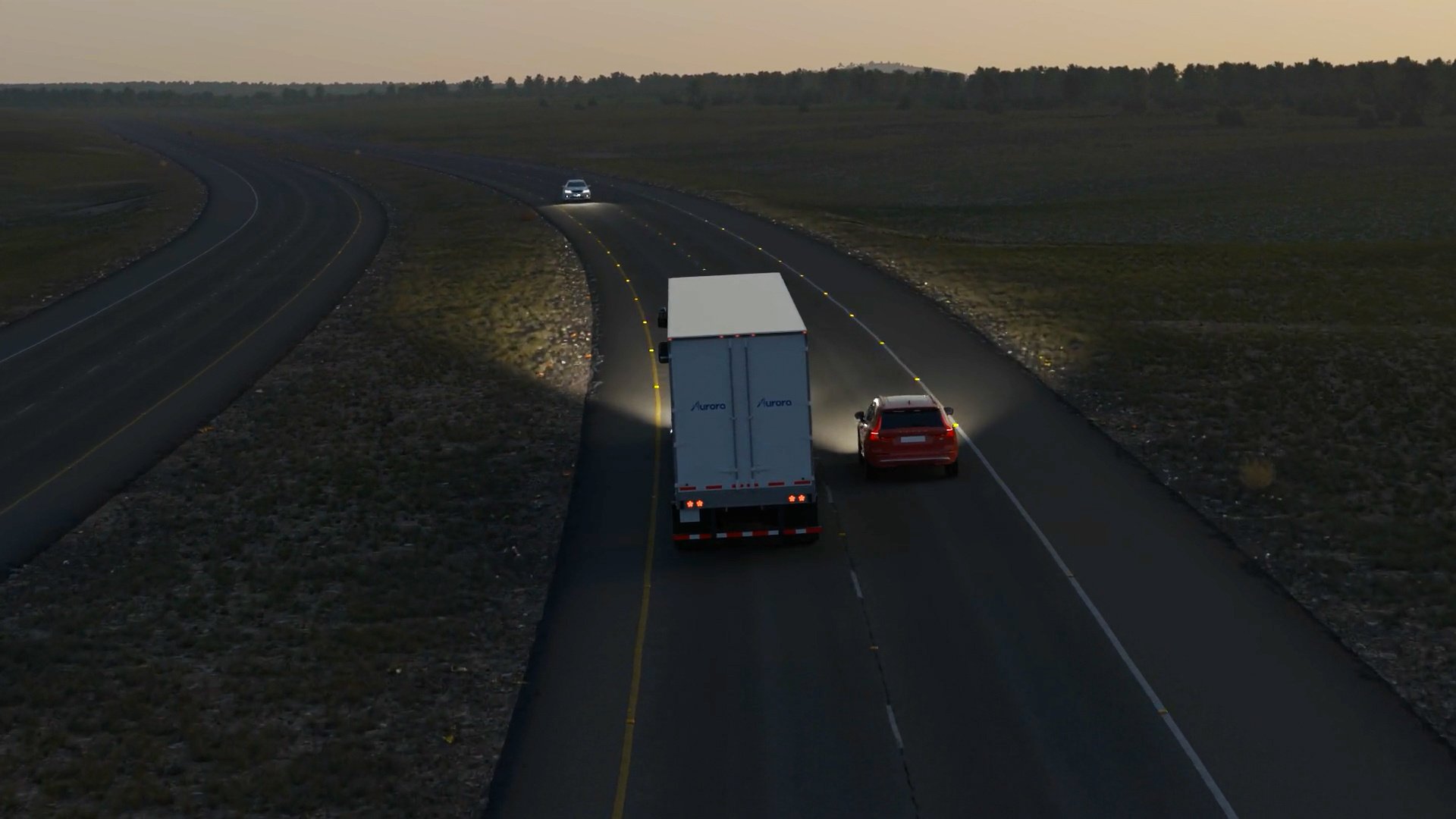
We’ve deployed our safety-focused self-driving technology in Texas, where it’s currently delivering loads in autonomy for customers on a daily basis. And soon, we’ll look westward to routes that connect our highways to major freight corridors in California.
Annually, California supports 2.4 billion tons of freight, largely distributed over its 50,000 miles of highway. As the Golden State’s economy grows, this number is expected to grow to 3.6 billion tons of freight by 2040. But this economic engine also comes at a terrible human cost — there are more than 400 fatal collisions in the state involving trucks per year. This is unacceptable.
The promise of self-driving — beyond its impact in strengthening supply chains and helping customers increase fleet utilization — lies in its value for improving road safety. This is core to our mission, and we’ve pledged to only launch our driverless product after we’ve closed our safety case.
A critical piece of that work is leveraging our industry-leading Virtual Testing Suite. Last year, we shared how Aurora leverages NHTSA’s detailed list of crash types and creates simulations to better understand how the Aurora Driver would handle challenging situations. Strong performance in these simulations helped show how we are preparing our product to safely navigate some of the toughest situations drivers face on the road.
Using learnings from this approach, Aurora examined fatal collisions involving human-driven vehicles on California’s I-5 — one of the United States’ most heavily used freight corridors and a crucial part of California’s freight ecosystem.
Learning from human drivers’ collisions in California
Aurora examined data of 14 fatal crashes on I-5 in Sacramento County between 2018-2022 and recreated those collisions in simulation to understand how the Aurora Driver would have acted if put into various positions in the scene.
For example, we simulated similar situations in which the Aurora Driver was the initiating or reacting vehicle in the collision scenario, or in place of different vehicles involved in the collision.
Our analysis found that, in these simulated scenarios, the Aurora Driver would not have caused any of the fatal collisions.
Applying learnings from simulation
Simulation is an essential piece of our development and validation process. It allows our autonomous trucks to experience the rarest of circumstances that might never be encountered over millions of miles of highway driving. High performance in simulation gives us the confidence to deploy the Aurora Driver to haul real commercial loads, and today our autonomous trucks drive thousands of miles for customers each week.
The following simulations show how the Aurora Driver can bring autonomy’s safety benefits to California. In these videos, other vehicles are represented by blue boxes and pedestrians are represented by smaller pink boxes:
Complicated Merge: In the original scenario, a passenger vehicle traveling in the right lane hit a pedestrian standing in the lane as they tended to a disabled vehicle parked on a narrow shoulder near an onramp. In our simulated scenario, the event is modified to represent an even more difficult scenario: The Aurora Driver takes the onramp and sees and tracks the pedestrian, slows down, and avoids them while negotiating a complex merge with a vehicle in an adjacent lane.
Reacting to a Collision Ahead: In the original scenario, a passenger vehicle collided with a stopped motorcycle and standing motorcyclist and then a truck caused a secondary collision when it was not able to avoid the motorcyclist in the lane of travel as a result of the first collision. In our simulated scenario, the Aurora Driver recognizes the initial collision and responds quickly — moving out of the way in time to prevent the secondary collision.
Learning from variations
A critical advantage the Aurora Driver has is that it can be trained on millions of difficult virtual driving scenarios before its tires ever hit the road. By building simulations in our Virtual Testing Suite, we can run the Aurora Driver through a wide range of scenarios — including thousands of permutations of a single scene. This allows us to adjust the speed, actors, weather, road infrastructure, and other parameters to test how our vehicle behaves.
We also leverage multiple types of simulation to help us better understand the Aurora Driver’s capabilities. The videos shown above highlight how we train the Aurora Driver to make decisions, and the video below features our Perception Simulation tool, which uses realistic recreations of the world to help validate our technology’s long-range perception.
Wrong-Way Driver: In the original incident, a three-way collision occurs due to a wrong-way driver on the highway. We iterated on the original incident in simulation by placing the Aurora Driver in the position of avoiding the wrong-way driver while accounting for a passenger vehicle alongside it in the adjacent lane. In our simulated scenario, the Aurora Driver’s long-range FirstLight lidar recognizes the wrong-way driver far in advance and is able to safely complete a contested merge to move out of the way — preventing the collision from occurring.
More than 5,000 fatal crashes involving large trucks occur on our nation’s highways annually. Now, as California policymakers consider the future of autonomous trucking in the state, we can’t afford to close the door on this technology. We need all the tools at our disposal, including autonomous trucks, to save lives and make our roads safer for everyone.
Disclaimer: Fatal collision scenarios were created in simulation using and varying details from police reports. While these simulations are generally representative of the type of original collision, details may differ between the original collision and the simulated scenario.
Delivering the benefits of self-driving technology safely, quickly, and broadly.