The Future of AI in Self-Driving: Facilitating and Benefitting from Scale
Engineering | From our leaders | September 12, 2024 | 4 min. read
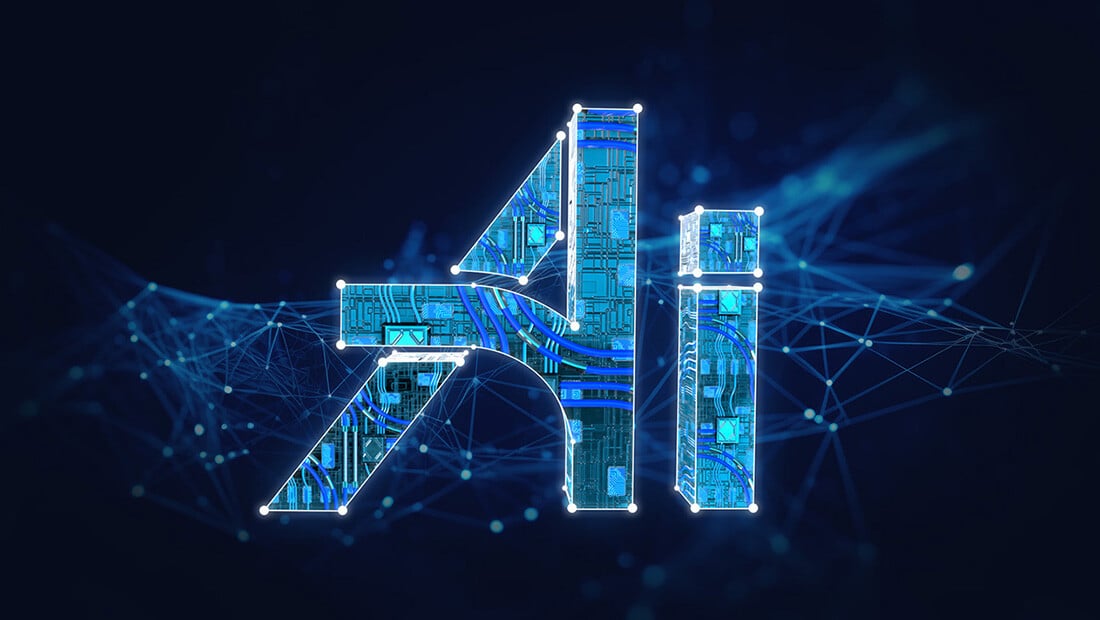
We founded Aurora nearly eight years ago to deliver the benefits of self-driving technology safely, quickly, and broadly. This mission has given purpose to our team and direction to our development: our product and our business are grounded in improving safety, creating value quickly, and scaling broadly.
Recently, we shared how we’ve leveraged advances in artificial intelligence to build a product that we can both verify safely and train quickly. Last week, I shared with our largest trucking customers how we’re building our product to deploy broadly: how we’ve specifically architected it to facilitate and benefit from scale.
Facilitating scale
Trucking logistics today is a vast, complex network that was built organically and evolved gradually into what is now a nearly $1 trillion dollar industry in the United States alone. Today, over 13 million trucks operated by more than 750,000 carriers and private fleets move over 30 million tons of goods across the country every day1. They do so on a range of roadways, between a variety of endpoints, and pulling a number of trailer types at a cost that clears the market for shippers and their customers.
To serve this industry at scale, a compelling self-driving system needs to not only be safe; it must also quickly adapt to shifting operating conditions, rapidly expand across new routes, endpoints, and trailer types, and do so at operating costs that allow it to serve the industry profitably.
Architecturally, we’ve made several choices to facilitate this expansion:
- Our Verifiable AI allows us to systematically introspect, specifically train, and concretely verify the Aurora Driver across a broad spectrum of operating conditions. This approach is more effective than a rules-based system and more efficient than an end-to-end monolith. It’s specifically designed to learn from experience and benefit from scale.
- Our virtual testing engine exposes our AI to roadways, scenarios, and conditions it has never seen before, at rates it may never see in practice. This early, expansive, and exacting exposure to new driving environments allows us to safely and efficiently train the Aurora Driver and validate its performance before truck rubber ever meets new road.
- Our multi-input sensor suite endows our AI with superhuman senses that it treats as complements, rather than dependencies. This treatment is important: when designing a multi-input system, one can make it dependent on all, or robust to any. For many years, strict dependency was assumed for self-driving systems: if any input fails or is inconsistent with the others, the thinking went, the system will fail. This is not how the Aurora Driver works. Instead, we’ve designed it to treat each input as complementary to – but not strictly necessary for – safe operation of the whole. If readings from a camera are unreliable, the Aurora Driver will lessen its reliance on them. If a radar, lidar, or other onboard system is obscured or damaged, the Aurora Driver will leverage complementary sensors to perform its mission safely. The practical effect of this approach is a product that is far more capable and robust than alternatives that depend on – and are more likely to fail with – a limited sensor suite.
- Our Fleet Intelligence technology gives each Aurora Driver-powered vehicle access to observations of all other such vehicles on the road. This system continuously consolidates, synthesizes, then disseminates observations, endowing every truck with the collective intelligence of the entire fleet. As with its onboard sensors, our AI has no hard dependency on its map being up to date or Fleet Intelligence being operational; when it encounters environments that don’t match what it expected to see, it bases its actions on its own observations and sends those to our Fleet Intelligence system for redistribution. In this architecture, the map and these observations serve not as a crutch, but as a networked sixth sense.
- And finally, we’ve designed our AI to continuously adapt to the unique weight and configuration of the trailer it’s pulling. Today, the Aurora Driver estimates these properties to confirm the information provided to it by the bill of lading or weigh station attendant. Ultimately, we expect this estimate to be able to serve as the system’s primary source for trailer weight information. The Aurora Driver’s controller, meanwhile, is designed to take both trailer configuration and weight information into account when making its decisions. As we deepen our penetration of open lanes, this adaptability will allow the Aurora Driver to operate a diverse range of trailers in service of more customers.
Structurally, we’ve assembled a broad ecosystem of partners to build, deploy, and operate Aurora Driver-powered trucks at large scale.
- No one is better suited to build and support self-driving trucks as reliably and extensively as major OEMs like Volvo and Paccar.
- No one is better equipped to manufacture and support highly-reliable self-driving hardware at scale than a leading Tier 1 supplier like Continental.
- And no one is better positioned to operate these self-driving trucks in service of the trillion dollar U.S. trucking industry than the carriers and fleets that have built and refined that operational muscle over decades.
Benefitting from Scale
As trucks developed, built, and deployed by this ecosystem proliferate across the U.S. highway network, their collective experience grows, the economies of scale they benefit from engage, and the network benefit they provide to customers expands. We’ve designed for this. By creating a product that efficiently acquires, processes, and learns from experiential data, we’ve set it up to benefit from three mutually-reinforcing flywheels:
- A growing fleet generates more data, accelerating capability expansion and driving adoption
- Larger production volumes drive down the cost of self-driving hardware, increasing its profitability, and accelerating adoption
- Large fleets cover more of the road network, increasing the network benefit they provide, and – you guessed it – driving further adoption
We expect our approach to AI and the data these flywheels generate to create a self-perpetuating advantage in a data-driven world. One that not only benefits our current customers, but ultimately, delivers the benefits of self-driving technology broadly across trucking, ride-hailing, delivery, and beyond.
To learn more about Aurora's approach to Verifiable AI, check out the rest of our series:
- Aurora's Verifiable AI Approach to Self-Driving
- AI Transparency: The Why and the How
- AI Alignment: Ensuring the Aurora Driver is Safe and Human-Like
1 https://www.trucking.org/economics-and-industry-data